Recently, one of the biggest convergences is between the fields of AI/ML and IoT, which enables the rise of Artificial Intelligence of Things (AIoT). AIoT combines data collected by sensors and other devices with the intelligence offered by AI/ML to enable real-time, intelligence on the edge. By converging AI/ML and IoT capabilities, AIoT solves two problems by merging them together into one solution. The goal of AIoT is to enable more efficient IoT operations, improve human-machine interactions and enhance how data is managed and analyzed.Â
Edge intelligence allows a high level of data to be processed and analyzed, and for decisions to be made locally, without being sent to the cloud. Take for example a self-navigating drone, instead of relying on a service hosted on the cloud to tell the drone where to go next, the drone itself is now able to decide its own path in the field, even when connections to cloud hosted services are not reliable.
The evolution of IoT into AIoT progressed in three distinct stages:Â
- Enabling core capabilities on the edge, including basic sensor development, integration with available devices, etc.
- Collecting the data generated from these sensors and storing them in a structured form on a central data store, typically on the cloud.
- Realizing the synergy between AI/ML and IoT and combining them together into AIoT.
In other words, while IoT provided access to a large base of information, AI/ML has brought in intelligence and decision making.
While great advancements toward AIoT have occurred, there are three main challenges in enabling intelligence on the edge to consider:
- Latency: When decision making occurred on the cloud, the only available option for an IoT device was to send the data (say, a captured image from a camera) to a service running on the cloud and get a selected decision from the service. This meant large volumes of data were transferred over slow or unreliable networks, resulting in delays–in many scenarios these delays could be extremely critical.
- Cost of computing and data transfer: Apart from the delay itself, the sheer volume of requests would greatly increase the cost of both transferring data back and forth and the cost of computing on the cloud–decreasing the feasibility of these implementations.
- Design divergence: While initial AI/ML solutions leveraged the cloud for vast amounts of computing power for training models, advances in the ability to move around or run a trained model (to calculate a decision) caused a divergence between the design of the solution and the hardware needed for the training and deciding components.
In parallel, AI/ML learning methods also evolved–these became a lot more efficient with regards to computing power usage, and the type of hardware needed for a machine to learn or to decide. Combining AI and IoT was the next logical stage in this evolution.
Design of an AIoT Solution
The physical components of an AIoT solution are not that different from a standard IoT implementation; the design of the AI/ML backend also does not change drastically. The changes are to a few specific parts of the core devices on the edge–that are now more powerful–and enable a huge range of flexibility with the scenarios that can be handled, and how solutions are designed using these devices.Â
Impact on Enterprises
Enterprises looking to benefit from AIoT may need to re-align roadmaps or make a few incremental changes based on their current state of adoption and based on the nature of their solutions.
For CXOs
There are four suggested, broad strategies for CXOs based on their level of investment today in the areas of IoT and AI/ML that are depicted in the following graphic.
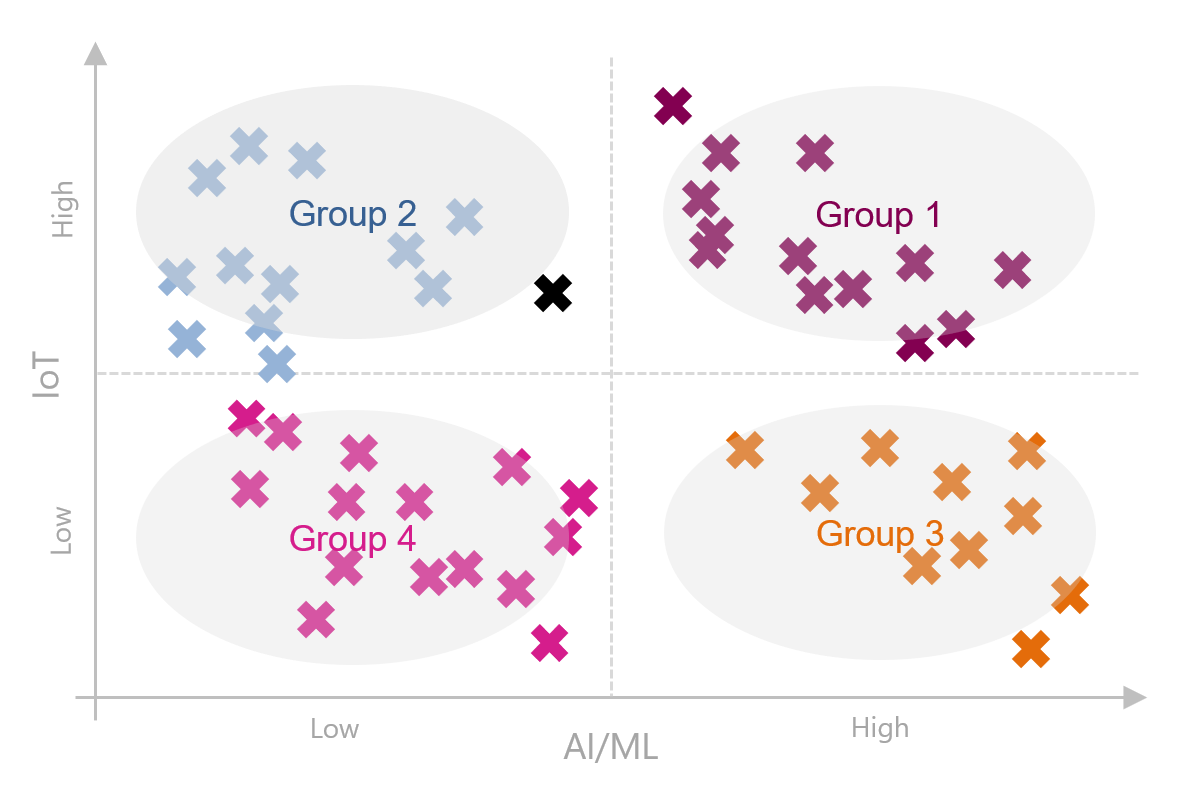
- Group 1: Current level of investment in AI/ML and IoT are both high. The best option for these enterprises would be to evaluate the current roadmaps to identify incremental additions/upgrades to edge devices. This would leverage the existing investment in AI/ML and at the same time start demonstrating benefits early without increasing risk.
- Group 2: Level of investment is higher in IoT compared to AI/ML. These enterprises stand to gain the most by shifting the ratio of investment toward AI/ML while making small changes to the device sets on the edge. They may also need to re-evaluate their current process to consider moving the decision part of the step to the edge.
- Group 3: Level of investment in AI/ML is higher than investments in IoT. These enterprises would probably need minimal re-alignment with their roadmaps–a quick look at the implementations of the steps that train the ML models would provide the list of actions to take–epics/stories that needed intelligence on the edge can move higher up in the product backlog.
- Group 4: These enterprises have the maximum freedom with the roadmap their implementations can take. Any product or platform roadmap planning can easily include AIoT epics and stories, with minimal impact to tasks already underway.
For Architects/Program Managers
For architects and program leads working on such initiatives within the company, it’s mainly a mindset change in regards to how the solution is designed, including capabilities of the devices on the edge and where the decision-making step in a process happens. Feasibility for scenarios such as the drone automatically calculating its own path instead of relying on a cloud-hosted service are now better than before, and a few demos or proof-of -concept attempts could now move many of these stories from the backlog and bring implementation dates forward.
Realistic Predictions for AIoT
While AIoT in its re-imagined, converged form may be new, the two original fields (AI and IoT) that merged to create AIoT are both mature and well into mainstream adoption. The benefits offered by AIoT for enterprises today, combined with the low risk involved with the small incremental changes outlined above and the cost savings that result in moving the decision making to the edge, create a lethal combination that positions AIoT to revolutionize business processes.
IDC has predicted that the global spend on IoT will reach $1.1 trillion in 2023, up from $726 billion in 2019. Moreover, IDC predicts there will be around 40 billion IoT devices by 2025, resulting in a significant influx of data that will make it increasingly challenging for enterprises to drive operational insights and optimize their analysis of the large sums of data available. AIoT can help solve this problem by bringing increased intelligence to data management and thorough analysis.Â
If it isn’t already, now is an ideal time for AIoT to be a top priority for C-suite IT leaders.